DeepL Blue Score Explained: What You Need to Know
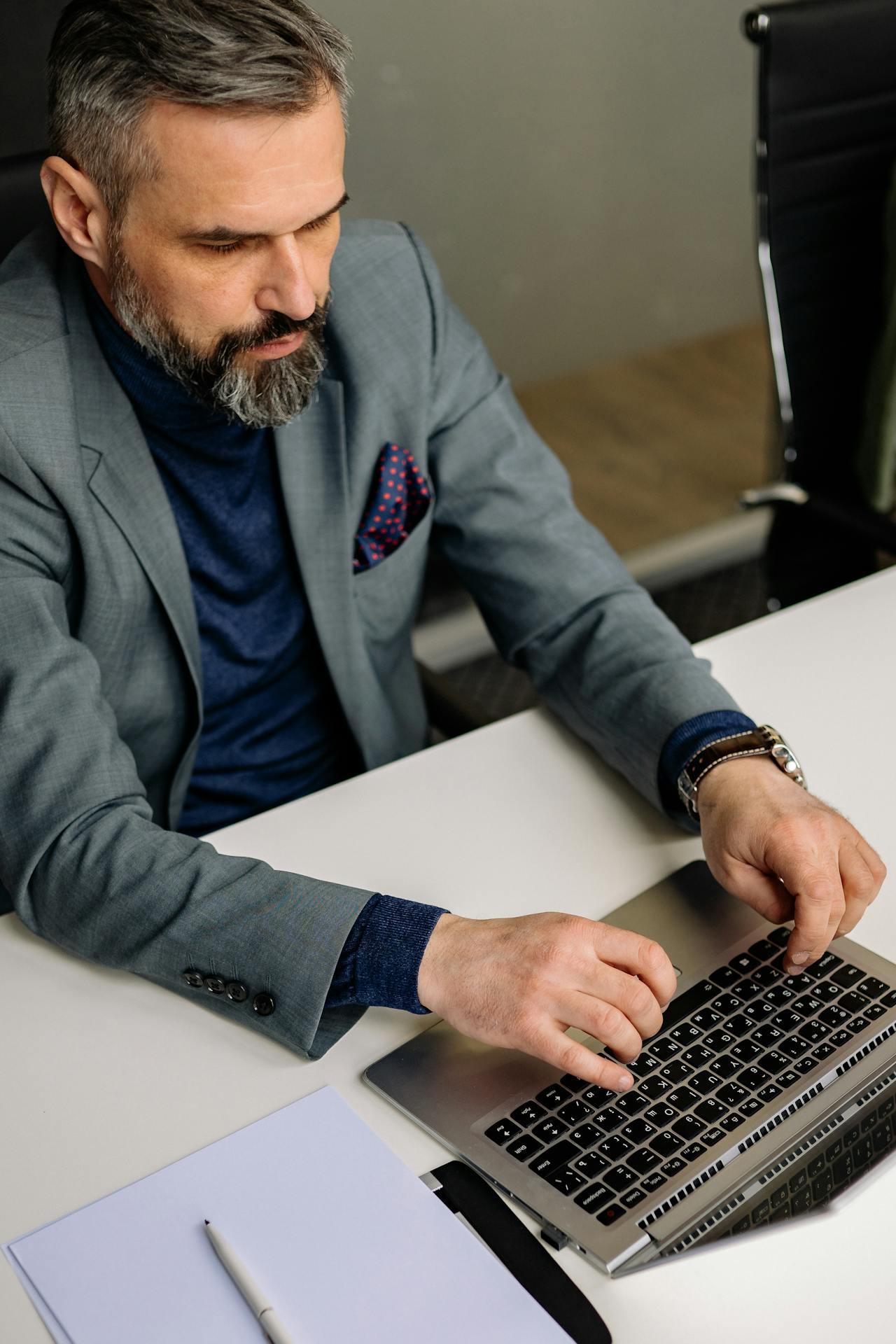
Understanding DeepL Accuracy
DeepL’s performance as a translation tool has garnered attention, particularly for its accuracy and user satisfaction. In this section, you’ll learn more about how users feel about DeepL’s services and the analysis of its Bleu score.
User Satisfaction with DeepL
User satisfaction is a vital metric when assessing the effectiveness of any translation service. According to reports, DeepL’s accuracy has been positively received by users, especially for European language pairs. The company has conducted experiments supporting these claims, affirming that many users prefer its services over other translation tools (Phrase).
Here are some statistics highlighting user satisfaction with DeepL:
Metric | Score |
---|---|
DeepL Bleu Score | 4.9 out of 5 |
Users that prefer DeepL | High, particularly in European language pairs |
This high score indicates that users are generally pleased with the translations provided by DeepL, further solidifying its reputation in the field of machine translation.
Analysis of Bleu Score
The Bleu score is an important measure used to evaluate the quality of machine translation. For DeepL, the Bleu score stands at an impressive 4.9 out of 5 (Word Spinner) which signifies a high level of user satisfaction. This score reflects the high accuracy of translations provided by DeepL and encourages users to depend on the platform for their translation needs.
DeepL’s Bleu score is particularly noteworthy when compared to its competitors, likely due to its focus on improving accuracy, customization, and data protection. Users also appreciate that DeepL allows for alternative translation options for specific words, enhancing the overall experience.
If you’re curious about how DeepL stacks up against other platforms, consider visiting our article on is there anything better than deepl? for a deeper insight into various translation tools.
Understanding these aspects of DeepL can help you determine whether it meets your translation needs, especially if accuracy is a top priority for your projects. You might also be interested in knowing is deepl actually accurate? or exploring how it relates to AI in our article on is deepl based on ai?.
Evaluating Translation Quality Metrics
When it comes to assessing the efficiency of translation systems like DeepL, understanding the metrics used in evaluation is crucial. Two major points of focus are the BLEU score and the comparison between DeepL and traditional machine translation systems.
Bleu Score: Strengths and Limitations
The BLEU score, which stands for “Bilingual evaluation understudy,” is a key metric used to evaluate machine-translated text. It works by comparing segments from machine translations to human reference translations, measuring the overlap in n-grams (a sequence of n items from a given sample) to ascertain translation quality. This method offers a quantifiable measure of how closely machine-generated text aligns with human standards.
Strengths of BLEU Score
- Rapid Feedback: BLEU scores provide quick insights into the quality of translations, making them useful for iterative improvements in machine translation systems.
- Comparison Capability: The scores allow for the evaluation of various machine translation systems in similar contexts, helping enterprises choose the best solutions.
Limitations of BLEU Score
While BLEU scores have their benefits, they also present several challenges:
- Context Ignorance: BLEU only rewards exact matches of n-grams, failing to account for synonyms or variations in word usage. This can lead to misleading evaluations of quality when meaningful translations are penalized unnecessarily (Towards Data Science).
- Language-Specific: The scores are tied to specific test sets and language pairs. Comparing BLEU scores across different languages can yield non-comparable results, making it essential to back these metrics with human assessments before validating a translation’s quality in production (ModernMT).
Feature | Strengths | Limitations |
---|---|---|
BLEU Score | Provides rapid feedback, allows for comparison of systems | Ignores context and meaning, can misrepresent quality across languages |
Feedback Utility | Supports iterative development for machine translation systems | Requires human judgment for reliable assessment |
DeepL vs. Traditional Machine Translation
DeepL is often compared to traditional machine translation systems due to its innovative approach and advanced AI technology. The differences in translation output can be quite significant.
- Translation Quality: DeepL typically produces translations that are considered to be more fluent and accurate compared to its competitors. The effectiveness of its AI model reflects in higher user satisfaction levels, as many users recognize its nuanced understanding of language (is deepl actually accurate?).
- Use of AI: Unlike traditional systems that rely primarily on rule-based approaches or more basic statistical methods, DeepL employs advanced neural networks. This difference leads to more context-aware translations, offering a more human-like understanding of language nuances (is deepl based on ai?).
- Feedback Loop: While traditional systems may lack the rapid adjustment capabilities, the continuous improvement processes of DeepL leverage feedback and translation data to enhance quality and adapt to user needs effectively. This positions DeepL as a modern choice in the field of translation technology.
Comparing DeepL with traditional systems can help you understand their distinct advantages. For more on the limitations of AI translations, including detection concerns, you might explore our article on can translated ai be detected?.
Through this evaluation of metrics and comparative analysis, you can better grasp how DeepL stands in the machine translation landscape and what those metrics mean for your translation tasks.